Exploring Recipe Auto-Learning: Revolutionizing Cooking Skills
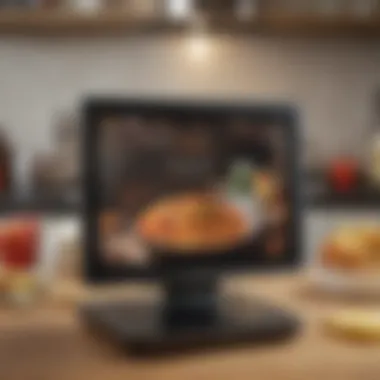
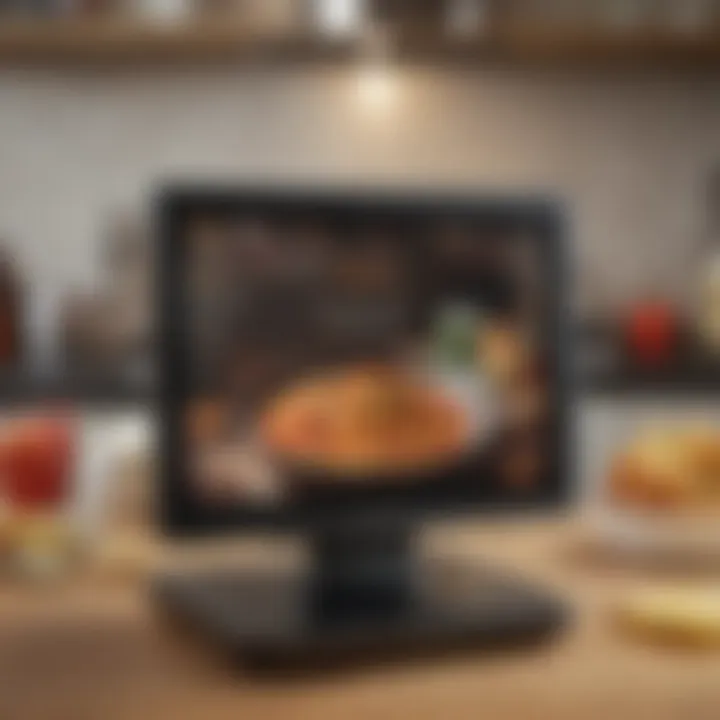
Intro
In today's fast-paced world, technology is entwined with every aspect of our lives, including the art of cooking. The emergence of recipe auto-learning marks a significant shift, merging culinary tradition with modern innovation. By embracing this evolving technology, culinary enthusiasts can elevate their cooking skills, experiment with flavors, and simplify meal prep. As we delve deeper into the various facets of recipe auto-learning, we will explore platforms and methods that redefine how cooking is experienced in home kitchens.
Ingredients Breakdown
Substantial knowledge of ingredients is crucial in refining culinary skills. An understanding of primary and optional ingredients can significantly influence the final dish. Below, we dissect these components.
Primary Ingredients
These are the backbone of any recipe. Quality is paramount here. Fresh herbs, seasonal vegetables, and high-quality proteins like chicken breasts contribute significantly to flavor and nutrition.
Optional Ingredients
Optional ingredients permit the cook to introduce variability. Things like spices, saucing agents or extra toppings enhance creativity. Variations whisper a secret—what if you did this instead of that?
Essential Kitchen Tools
Having the right tools can transform cooking from mundane to exciting. Some must-have basics include:
- Chef's knife
- Cutting board
- Non-stick skillet
- Measuring cups and spoons
Equipping your kitchen with these items opens a path to successful meal preparation.
Step-by-Step Preparation
Following a systematic method ensures greater efficiency in cooking. Here’s how you can streamline the process.
Prepping the Ingredients
Preparation is critical. Start by washing, chopping or marinating ingredients. This can prevent confusion later in the cooking process. Misplacing even one ingredient may result in an imbalanced dish.
Cooking Techniques and Methods
Master various techniques, from sautéing to slow cooking. Through practice and recipe auto-learning functionalities, become familiar with the best method for each ingredient.
Assembly and Presentation Tips
How a dish appears influences palatability. Colors, textures, and garnish make meals visually appealing. Breathe more life into your dishes with simple plating techniques.
Dietary Considerations
Dietary flexibility is vital in today’s culinary practice. Below are various components to account for.
Gluten-Free Options
For those avoiding gluten, alternatives exist like quinoa flour and almond flour, which serve as substitutes in mainstream recipes.
Vegetarian and Vegan Substitutes
Replacing meats or dairy is easily achievable. Ingredients such as tofu, chickpeas, and plant-based milk can fit into many recipes without a hitch.
Nutrition Facts & Nutritional Considerations
Understanding nutritional content enhances the preparation of balanced meals, leading to healthier choices and better satisfaction with one’s diet.
Variations and Customizations
Creativity truly shines in this section. Alter structures enhance engagement with food.
Flavor Enhancements
Add flavor through herbs, spices, or acidic components like lemon. Small adjustments can bring depth to any recipe, transforming ordinary into extraordinary.
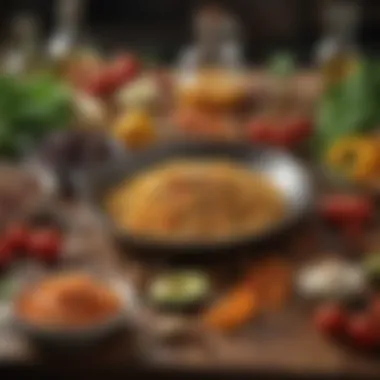
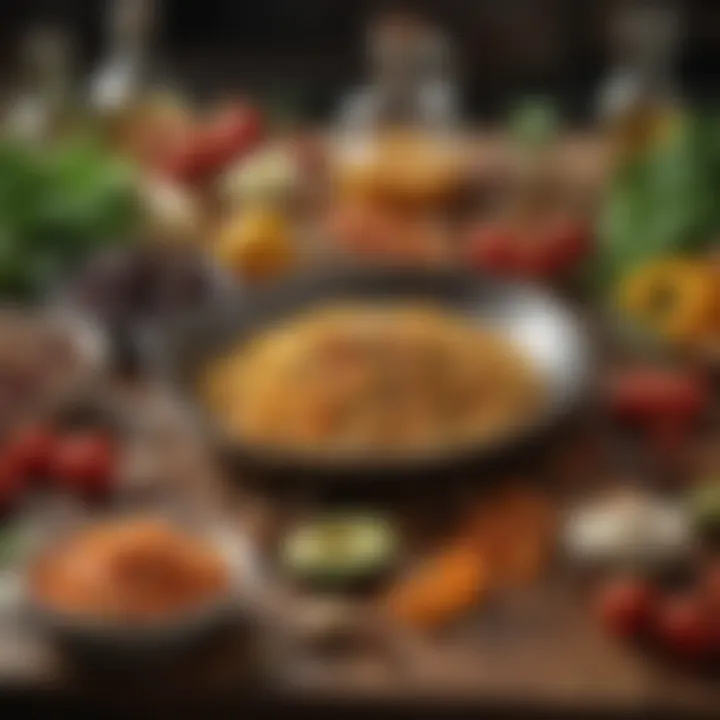
Alternative Cooking Methods
Experiment with different preparation styles. Grilling, steaming, or baking can introduce distinct textures and flavors.
Pairing Suggestions (Sides, Drinks, etc.
) Complement dishes thoughtfully. Each main needs suitable accompaniments to enhance the entire dining experience.
Common Questions and Answerss and Troubleshooting
Frequently Asked Questions
Modern cooks often face challenges. Familiarizing oneself with common issues can pave paths to success.
Common Mistakes to Avoid
Watch for mismeasured ingredients or inadequate temperature settings, as these commonly derail attempts at perfection.
Solutions to Potential Problems
Use tech-driven recipe auto-learning systems that offer troubleshooting tips. These offer guidance ranging from selecting appropriate methods to ingredient substitutes, streamlining the cooking process.
Recipe auto-learning systems will shape the culinary landscape, embracing creativity while refining skills.
Engagement with recipe auto-learning stands to markup boundaries in the kitchen. Optimize this technology to redefine your cooking experience.
Understanding Recipe Auto-Learning
In today's fast-paced world, cooking often seems like a time-consuming task. Yet, the integration of technology into cooking practices has opened a new realm where learning and skill enhancement is streamlined. This is the essence of Recipe Auto-Learning. It redefines the culinary experience by enabling cooks—both novice and experienced—to optimize their cooking processes, experiment with new recipes, and manage their kitchens more efficiently.
Through effective automation, chefs can save substantial time while improving their culinary skills. By analyzing individual preferences and dietary needs, Recipe Auto-Learning fosters personalized solutions which elevate the cooking experience. It transforms the way meal preparations are approached and encourages creativity in the kitchen.
Defining Recipe Auto-Learning
Recipe Auto-Learning refers to the application of technology in recipes management and meal preparation. It utilizes artificial intelligence and machine learning to derive insights from culinary data. With the development of these technologies, auto-learning systems can suggest, create, and modify recipes based on existing ingredients, cooking skill levels, and even dietary restrictions.
Users input their preferences, and the auto-learning system adapts accordingly. Over time, as users apply the suggestions, the system learns and evolves based on feedback. This captures a unique fusion of skill development and cooking, allowing for a constant touch of innovation and relevance in every meal someone prepares.
Historical Context of Auto-Learning in Cooking
The historical context of Auto-Learning in cooking unfolds a narrative of relatively recent advancements in culinary methodology, driven largely by technological progress. Early forms of recipe management were limited to cookbooks, with little alignment to personal tastes or usage patterns. The programming languages and computer technologies from the late 20th century began the journey towards automated systems.
In the early 2000s, many cooking blogs and websites emerged, enabling the first digital exploration of recipes. The limitations of searching for specific dishes based only on titles and ingrained categorizations inspired further innovations in algorithm-based approaches. With the advent of machine learning in the 2010s, it then became increasingly feasible to analyze larger datasets consisting of cross-referenced recipes, preferences, and ingredients available.
The culmination of these developments has resulted in systems from companies like Yummly or Cookpad that effectively help to interpret vast repositories of culinary knowledge and present it coherently to the user. These shifts depict dramatic progress towards a highly personalized cooking space made easier through advanced technology.
The Technology Behind Recipe Auto-Learning
Recipe auto-learning is deeply interwoven with various technological frameworks. These frameworks aid in gathering culinary data, processing that information, and generating actionable recipe content. The resulting designs create an intersection between culinary arts and technological advancement, contributing significantly to modern cooking. They reshape cooking traditions and it gives food lovers the chance to elevate their culinary pursuits.
Machine Learning Basics
Machine learning is the foundational layer that powers recipe auto-learning systems. It involves an infrastructure where algorithms identify patterns and glean insights from data. By analyzing painstakingly compiled databases, the algorithms can build predictive models that suggest recipes based on past success rates. Yes, a dish you previously cooked could influence what recommendations arise next!
Different forms of machine learning come into play, from supervised learning—which requires labeled data—to unsupervised methods that find patterns without those predetermined labels. Between these two, reinforcement learning demonstrates proficiencies through trial and error as model adjust to responses from individuals. Thus, it lays the groundwork for well-informed culinary guidance.
Data Collection and Recipe Analysis
Data plays a critical role in how machine learning thrives. The operation of recipe auto-learning systems relies on meticulous data collections, often sourced from myriad platforms. Understanding which ingredients work, how taste interplays with cooking methods, and current trends forms an elemental understanding.
For the analysis part, the importance of structured data cannot be overstated. Structured recipes include ingredients, cooking times, and technique breakdowns that allow algorithms to find synchronicity between recipes.
It is crucial, however, to note that raw data can be an exhibitor of disarray. Ingredient substitutions and varying techniques across different cultures must be acknowledged. When the data quality is sufficient, it paves the way for efficient curriculum adjustments within recipe generation.
Algorithm Types Used in Recipe Generation
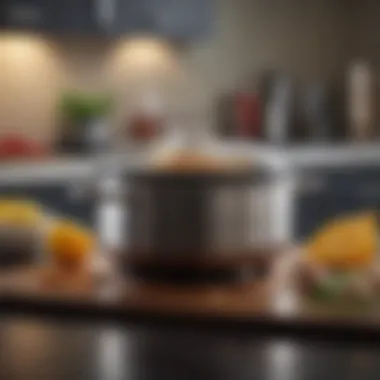
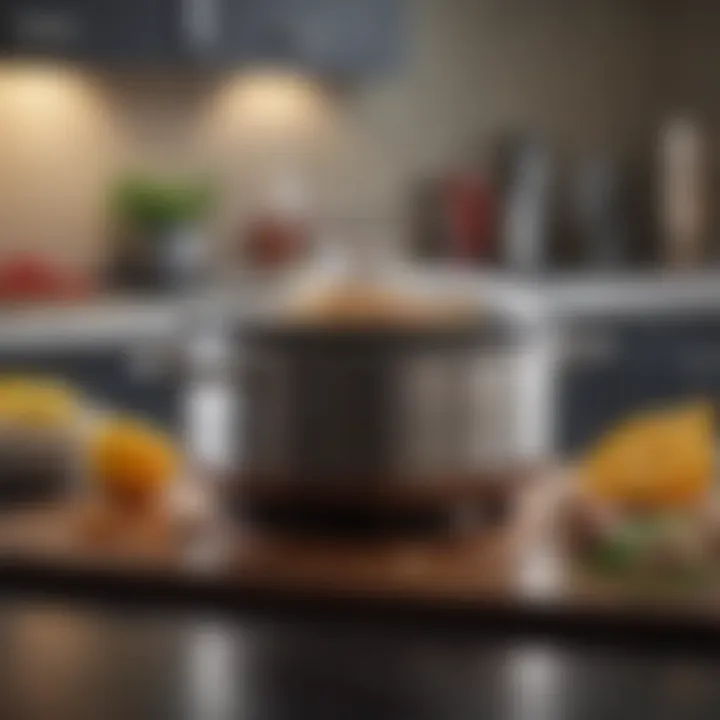
In creating recipes through technological means, diverse algorithms serve as the architects. The traditional algorithms rely primarily on decision trees and collaborative filtering, fetching suggestions based on similarities to prior user choices. In contrast, generative models offer innovative angles. These styles serve uncharted domains by conjuring new combinations that attract the intuitions of the culinary innovators.
Three key categories of algorithms include:
- Content-Based Systems: These increase recipe attractiveness by evaluating the characteristics of dishes previously enjoyed, producing variations tailored to preferences.
- Collaborative Filtering: Systems in this segment suggest recipes grasping common features enjoyed by a community rather than relying solely on individual data.
- Generative Models: These systems highlight creativity, synthesizing completely novel recipes. Limoncello-infused pasta, merging savory and sweet harmonies may be a visualized outcome.
As the technological landscape evolves, the shifts in these algorithms indicate promising directions toward greater personalization and efficiency. Self-adapting machine learning models present pathways to not merely replicate but innovate culinary experiences while catering to the basic needs of gastronomes.
Benefits of Recipe Auto-Learning Systems
Recipe auto-learning technology offers multiple advantages for both novice and experienced cooks. As this approach merges culinary skills with artificial intelligence, it opens new avenues for enhancing the cooking experience. This section highlights the significant elements of recipe auto-learning systems and their relevance in modern kitchens.
Time Efficiency in Meal Preparation
One of the most compelling benefits of recipe auto-learning systems is the time efficiency they provide in meal preparation. When пользователи rely on these platforms, they can quickly and easily access recipe suggestions that match their ingredients and cooking times. This is particularly useful in our fast-paced lives. People often struggle to find suitable meals that fit into their schedules.
Using these technologies, a home chef can get suggestions in a matter of seconds. No more flipping through cookbooks or endless scrolling on websites to find the right recipe. Furthermore, recipe auto-learning can streamline your workflow. For instance, platforms calculate cooking times and organize steps in a way that maximizes efficiency.
"Time is one component everyone values. Cooking technology can transform the arduous task into an effortless endeavor."
Socio-cultural changes demand fast solutions. Meal prep also becomes enjoyable, enjoying the benefits of organized and well-timed episodes of cooking, rather than haphazard trial and error.
Personalized Recipe Recommendations
Personalization is another strength of recipe auto-learning systems. Users often have unique dietary preferences, whether for health, ethical, or taste reasons. It can be annoying to sift through generic recipes that do not align with one’s required preferences or restrictions. Recipe auto-learning tackles this issue. These systems analyze user data and preferences, providing tailored suggestions.
By learning from previous interactions, the algorithms adapt over time to serve up recipes that individuals genuinely want to cook and eat. This leads to not just a better cooking experience but also encourages experimentation. Chefs may discover new flavors and techniques they might otherwise have ignored.
When looking for wholesome meals, people with specific dietary requirements benefit immensely from AI. Seeds and synergies of flavor revealed make the interaction not just informative but enlightening.
Reduction of Food Waste
Finally, there is a crucial and often overlooked benefit of recipe auto-learning: the reduction of food waste. With traditional cooking practices, it is common to stock more ingredients than needed. Often, these expire or go to waste. Recipe auto-learning assists users in utilizing their existing pantry items effectively.
Here are some points on how it achieves this:
- Systems can suggest recipes employing remaining ingredients in the kitchen.
- Users are encouraged to buy specific vegetables or proteins based on unused items, while focusing only on essentials.
- Adjusting portion sizes promotes mindful cooking and reduces incidents of unconsumed leftover food.
Preventing waste is increasingly vital in today's environmental age. Technologies like this bring attention to mindful eating while also twice as beneficial for budget considerations.
In summary, the benefits of recipe auto-learning systems span time efficiency, personalized recommendations, and diminished food waste. All of these targets appeal to the common problems encountered in modern cooking. Technological advancements, surprisingly, come through to enable a culinary evolution.
Challenges and Limitations of Recipe Auto-Learning
Recipe auto-learning, while promising, faces significant challenges that can affect its implementation and user acceptance. Understanding these hurdles is crucial for anyone looking to integrate this technology into their culinary practice. Key concerns revolve around data quality, cultural richness, and users' trust in technology.
Data Quality Concerns
One of the primary challenges in recipe auto-learning is data quality. Machine learning models rely heavily on the data fed into them. When the data is inaccurate, incomplete, or biased, the outcomes can also be far from ideal.
- Sources of data: Recipes sourced from online platforms may suffer from inconsistent measurements or ingredient lists.
- Variability in preparations: Different cooks follow varying techniques and interpretations, leading to further data distortion.
- Reliability checks: Ensuring the data's integrity requires rigorous checks and validations, which can be time-consuming.
To build effective and trustworthy auto-learning systems, achieving high-quality data is paramount. If technology produces subpar recommendations, it can frustrate users instead of aiding them.
Cultural and Regional Influences on Recipes
Recipes often reflect the cultural and regional. They cater specifically to tastes, traditions, and available ingredients of that location. Therefore, a significant limitation in recipe auto-learning arises when platform algorithms disregard these subtleties.
- Cultural dishes: Traditional recipes might not accurately represent local flavors when filtered through generic algorithms.
- Seasonality and habit: Regionally popular ingredients fluctuate based on seasons. Auto-learning must account for these changes.
- User diversity: There is a pressing need for inclusivity. A one-size-fits-all recipe could alienate users accustomed to specific meals.
For users, cooking isn't merely a mundane activity. It's a source of cultural pride. Failure to acknowledge these influences hinders the effectiveness of recipe auto-learning models.
User Acceptance and Trust in Technology
Building trust in recipe auto-learning systems is a challenge that technology developers must confront. Users need to feel confident in the results generated by these systems to adapt their cooking practices.
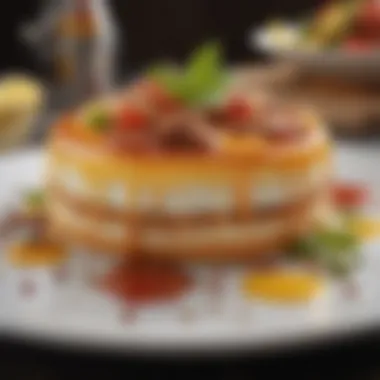
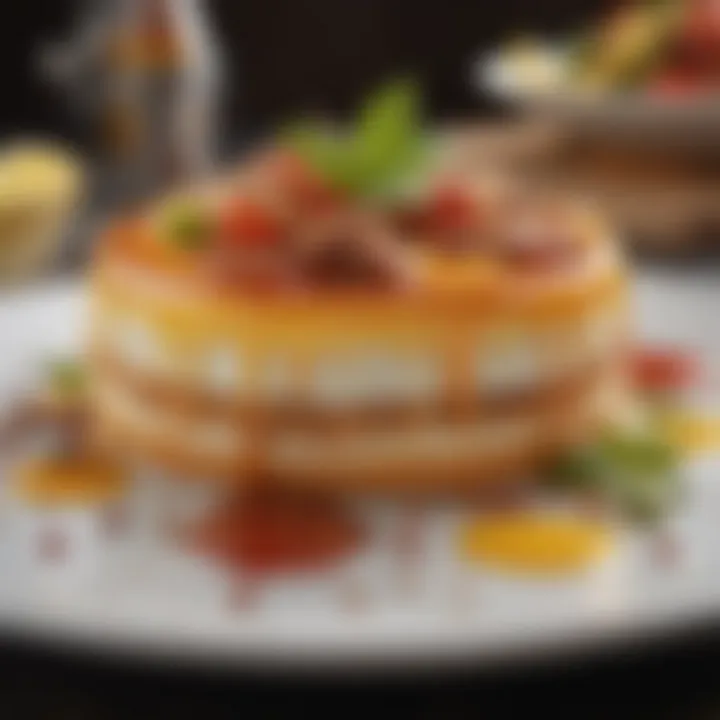
- Learning curve: Some users might struggle to navigate auto-learning applications. Complex interfaces or unclear functionalities can be off-putting.
- Skepticism about AI: As artificial intelligence becomes ubiquitous, users show concern over automation replacing traditional cooking methods. This skepticism can hinder user adoption.
- Demonstrating value: For users to engage regularly, platforms must demonstrate clear advantages over traditional methods. They should highlight significant improvements in ease and creativity.
For the culinary technology landscape to thrive, developers must focus not just on functionality but also on user connections.
It is crucial to confront the challenges of recipe auto-learning if we intend for technology and culinary creativity to intertwine effectively.
Real-World Applications of Recipe Auto-Learning
Recipe auto-learning systems transform how individuals approach cooking, illustrating significant potential in today’s expanding culinary landscape. These systems offer diverse applications that not only streamline meal preparation but also encourage creativity and personalized cooking experiences. From mobile applications to smart kitchen integrations, the ways this technology is applied showcases its relevance in modern cooking.
Consumer Applications: Apps and Platforms
Apps actively using recipe auto-learning have grown in popularity. These applications enhance user experience by offering targeted and personalized recipe suggestions based on user preferences and dietary restrictions. For example, apps like Yummly and Whisk utilize machine learning algorithms to suggest recipes that are tailored to individual tastes, frequency of cooking, and seasonal ingredients. Users can input what they have in their pantry, and the application efficiently scours its database for possible recipes.
Benefits of these applications include:
- Convenience: Quick access to varied recipes, reducing time spent searching
- Personalization: Individual meal planning that reflects personal taste and dietary requirements
- Stock Management: Helps to minimize food waste by utilizing available ingredients
- Knowledge Expansion: Encourages home cooks to experiment with unfamiliar cuisines and flavors
Applications enhance culinary skills by teaching users new techniques and fostering a more dynamic cooking environment. Those curious about data privacy must note that many apps require access to user behavior patterns to optimize recommendations.
Integration with Smart Kitchen Appliances
Smart kitchen appliances represent another realm where recipe auto-learning technology flourishes. Devices such as Instant Pots, smart ovens, and refrigerators now come equipped with functionalities powered by AI. These appliances can suggest cooking times and set temperatures based on user-selected recipes or fridge contents.
The integration works seamlessly, offering great management of cooking processes. With these smart appliances, cooks can start their machines remotely or receive step-by-step cooking guidance from their smartphones. This integration simplifies meal preparation greatly and ensures consistency in cooking results.
Some critical advantages include:
- Automation: Minimal advancement needed from the user once cooking inputs are selected.
- Consistency: Ensures meals are prepared to the same quality on multiple occasions.
- Safety Features: Smart devices can alert users to mistakes or hazards, like overcooking.
"Recipe auto-learning technology enriches how individuals cook, transforming the kitchen into a modern ecosystem of possibilities."
Future Trends in Recipe Auto-Learning
The realm of recipe auto-learning continues to evolve. As technology advances, it increasingly incorporates various methodologies and techniques designed to enhance culinary practice. Future trends in this area hold considerable significance, impacting how chefs, food enthusiasts, and home cooks approach their culinary experiences. By analyzing these trends, we can better understand technological integration into cooking and its implications for the kitchen environment.
Advancements in AI and Machine Learning
Advancements in artificial intelligence and machine learning are central to the future of recipe auto-learning. Modern algorithms analyze vast data sets related to recipes, cooking techniques, and ingredient combinations. Machine learning platforms refine recommendations over time, learning preferences and refining suggestions based on previous choices.
Hyper-personalization is set to take center stage. As algorithms leverage enhanced data on user dietary restrictions and tastes, the resulting recommendations become more aligned with individual needs. This not only aids in meal preparation but also promotes creativity in cooking.
Here are several noteworthy advancements to look for:
- Enhanced machine learning algorithms that consider health and nutrition alongside traditional flavor profiles.
- More sophisticated user interfaces with visually rich layouts making recipe browsing more intuitive.
- Better integration with wearable devices, allowing real-time tracking of dietary intake and tailoring recipes on the go.
“With improvements in AI, recipe auto-learning will provide solutions that fit lifestyles in ways we have not seen before.”
Predicted Changes in Consumer Behavior
As technology permeates daily routines, predicted changes in consumer behavior reflect growing engagement with innovative culinary solutions. Consumers are gradually shifting toward efficiency and sustainability. This behavior suggests a heightened preference for prepared meal kits and guided cooking experiences. Recipe auto-learning could cater to these preferences exceptionally well.
Factors influencing consumer behavior in the culinary space include:
- Increased demand for nutritional simplicity, leading to fewer ingredient options or preparation steps in auto-generated recipes.
- Willingness to experiment with global cuisine due to easier access to information and recipe generation methods.
- Anticipation for time-saving technologies, promoting culinary experiences that fit busy schedules without sacrificing flavor or quality.
Based on these insights, it seems that the trajectory of recipe auto-learning is squarely aimed at user agency in food choices cherished by the community. With these advancements, a harmonious relationship between culinary creativity and technology becomes increasingly evident. This balance outlines a future that invites every aspiring cook to cultivate their skills and expressions in the kitchen innovatively.
Concluding Thoughts on Recipe Auto-Learning
The Importance of Adapting to Technological Changes
Adapting to technological changes is not an option but a necessity in today’s fast-paced culinary environment. As digital tools evolve, cooks of all levels must embrace these advancements to enhance their skills. Recipe auto-learning provides a unique opportunity to save time, improve creativity, and refine cooking techniques.
Many culinary enthusiasts are familiar with traditional cooking methods. Yet, integrating technology can redefine what is achievable in the kitchen. As one employs recipe auto-learning, skills can flourish by leveraging the analytical capabilities of machine learning. This approach analyzes large datasets of recipes to suggest ways to improve, which can hasten the learning curve for both new and experienced cooks. The collaboration of culinary practice and tech helps in grasping not just how to prepare dishes but why certain techniques work. This understanding is fundamental for anyone looking to refine their culinary abilities.
Encouraging a Culinary Landscape That Collaborates with Technology
A collaborative environment between technology and culinary arts holds great potential for the future. This partnership not only inspires innovation but also fosters community engagement. As home cooks and professionals alike focus on sharing and enhancing their culinary practices through recipe auto-learning, they contribute to a collective journey toward gastronomic excellence.
While innovations continue to emerge, the essential aspect of peer support in the culinary world remains crucial. Enthusiasts can find not just data but a vibrant community willing to exchange ideas and techniques. Some platforms allow users to share their innovative uses of recipe auto-learning. The incorporation of cutting-edge tools, fluid communication among cooks, and a willingness to explore create a landscape ripe for culinary adventure and evolution.